Top 10 Data & AI Trends for 2025
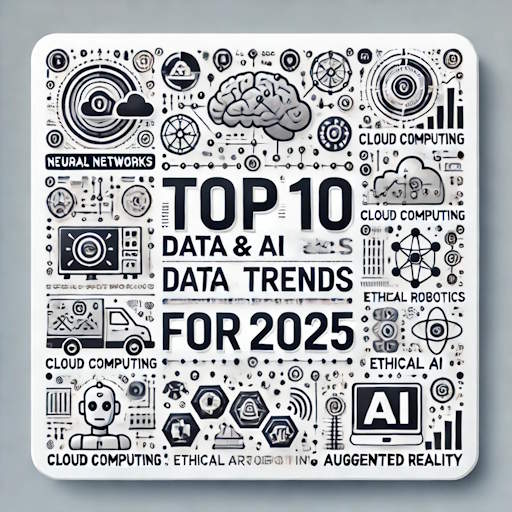
According to industry experts, 2024 was destined to be a banner year for generative AI. Operational use cases were rising to the surface, technology was reducing barriers to entry, and general artificial intelligence was obviously right around the corner. So… did any of that happen? Well, sort of. Here at the end of 2024, some of those predictions have come out piping hot. The rest need a little more time in the oven (I’m looking at you general artificial intelligence).
Here’s where leading futurist and investor Tomasz Tunguz thinks data and AI stands at the end of 2024 — plus a few predictions of my own.
2025 data engineering trends incoming.
1. We’re living in a world without reason (Tomasz)
Just three years into our AI dystopia, we’re starting to see businesses create value in some of the areas we would expect — but not all of them. According to Tomasz, the current state of AI can be summed up in three categories.
1. Prediction: AI copilots that can complete a sentence, correct code errors, etc.
2. Search: tools that leverage a corpus of data to answer questions
3. Reasoning: a multi-step workflow that can complete complex tasks
While AI copilots and search have seen modest success (particularly the former) among enterprise orgs, reasoning models still appear to be lagging behind. And according to Tomasz, there’s an obvious reason for that.
Model accuracy.
As Tomasz explained, current models struggle to break down tasks into steps effectively unless they’ve seen a particular pattern many times before. And that’s just not the case for the bulk of the work these models could be asked to perform.
“Today…if a large model were asked to produce an FP&A chart, it could do it. But if there’s some meaningful difference — for instance, we move from software billing to usage based billing — it will get lost.”
So for now, it looks like its AI copilots and partially accurate search results for the win.
2. Process > Tooling (Barr)
A new tool is only as good as the process that supports it.
As the “modern data stack” has continued to evolve over the years, data teams have sometimes found themselves in a state of perpetual tire-kicking. They would focus too heavily on the what of their platform without giving adequate attention to the (arguably more important) how.
But as the enterprise landscape inches ever-closer toward production-ready AI — figuring out how to operationalize all this new tooling is becoming all the more urgent.
Let’s consider the example of data quality for a moment. As the data feeding AI took center-stage in 2024, data quality took a step into the spotlight as well. Facing the real possibility of production-ready AI, enterprise data leaders don’t have time to sample from the data quality menu — a few dbt tests here, a couple point solutions there. They’re on the hook to deliver value now, and they need trusted solutions that they can onboard and deploy effectively today.
As enterprise data leaders grapple with the near-term possibility of production-ready AI, they don’t have time to sample from the data quality menu — a few dbt tests here, a couple point solutions there. They’re already on the hook to deliver business value, and they need trusted solutions that they can onboard and deploy effectively today.
The reality is, you could have the most sophisticated data quality platform on the market — the most advanced automations, the best copilots, the shiniest integrations — but if you can’t get your organization up and running quickly, all you’ve really got is a line item on your budget and a new tab on your desktop.
Over the next 12 months, I expect data teams to lean into proven end-to-end solutions over patchwork toolkits in order to prioritize more critical challenges like data quality ownership, incident management, and long-term domain enablement.
And the solution that delivers on those priorities is the solution that will win the day in AI.
3. AI is driving ROI — but not revenue (Tomasz)
Like any data product, GenAI’s value comes in one of two forms; reducing costs or generating revenue.
On the revenue side, you might have something like AI SDRS, enrichment machines, or recommendations. According to Tomasz, these tools can generate a lot of sales pipeline… but it won’t be a healthy pipeline. So, if it’s not generating revenue, AI needs to be cutting costs — and in that regard, this budding technology has certainly found some footing.
“Not many companies are closing business from it. It’s mostly cost reduction. Klarna cut two-thirds of their head count. Microsoft and ServiceNow have seen 50–75% increases in engineering productivity.”
According to Tomasz, an AI use-case presents the opportunity for cost reduction if one of three criteria are met:
- Repetitive jobs
- Challenging labor market
- Urgent hiring needs
One example Tomasz cited of an organization that is driving new revenue effectively was EvenUp — a transactional legal company that automates demand letters. Organizations like EvenUp that support templated but highly specialized services could be uniquely positioned to see an outsized impact from AI in its current form.
4. AI adoption is slower than expected — but leaders are biding their time (Tomasz)
In contrast to the tsunami of “AI strategies” that were being embraced a year ago, leaders today seem to have taken a unanimous step backward from the technology.
“There was a wave last year when people were trying all kinds of software just to see it. Their boards were asking about their AI strategy. But now there’s been a huge amount of churn in that early wave.”
While some organizations simply haven’t seen value from their early experiments, others have struggled with the rapid evolution of its underlying technology. According to Tomasz, this is one of the biggest challenges for investing in AI companies. It’s not that the technology isn’t valuable in theory — it’s that organizations haven’t figured out how to leverage it effectively in practice.
Tomasz believes that the next wave of adoption will be different from the first because leaders will be more informed about what they need — and where to find it.
Like the dress rehearsal before the big show, teams know what they’re looking for, they’ve worked out some of the kinks with legal and procurement — particularly data loss and prevention — and they’re primed to act when the right opportunity presents itself.
The big challenge of tomorrow? “How can I find and sell the value faster?”
5. Small data is the future of AI (Tomasz)
The open source versus managed debate is a tale as old as… well, something old. But when it comes to AI, that question gets a whole lot more complicated.
At the enterprise level, it’s not simply a question of control or interoperability — though that can certainly play a part — it’s a question of operational cost.
While Tomasz believes that the largest B2C companies will use off the shelf models, he expects B2B to trend toward their own proprietary and open-source models instead.
“In B2B, you’ll see smaller models on the whole, and more open source on the whole. That’s because it’s much cheaper to run a small open source model.”
But it’s not all dollars and cents. Small models also improve performance. Like Google, large models are designed to service a variety of use-cases. Users can ask a large model about effectively anything, so that model needs to be trained on a large enough corpus of data to deliver a relevant response. Water polo. Chinese history. French toast.
Unfortunately, the more topics a model is trained on, the more likely it is to conflate multiple concepts — and the more erroneous the outputs will be over time.
“You can take something like llama 2 with 8 billion parameters, fine tune it with 10,000 support tickets and it will perform much better,” says Tomasz.
What’s more, ChatGPT and other managed solutions are frequently being challenged in courts over claims that their creators didn’t have legal rights to the data those models were trained on.
And in many cases, that’s probably not wrong.
This, in addition to cost and performance, will likely have an impact on long-term adoption of proprietary models — particulary in highly regulated industries — but the severity of that impact remains uncertain.
Of course, proprietary models aren’t lying down either. Not if Sam Altman has anything to say about it. (And if Twitter has taught us anything, Sam Altman definitely has a lot to say.)
Proprietary models are already aggressively cutting prices to drive demand. Models like ChatGPT have already cut prices by roughly 50% and are expecting to cut by another 50% in the next 6 months. That cost cutting could be a much needed boon for the B2C companies hoping to compete in the AI arms race.